the Creative Commons Attribution 4.0 License.
the Creative Commons Attribution 4.0 License.

Understanding summertime peroxyacetyl nitrate (PAN) formation and its relation to aerosol pollution: insights from high-resolution measurements and modeling
Baoye Hu
Naihua Chen
Rui Li
Mingqiang Huang
Youwei Hong
Lingling Xu
Xiaolong Fan
Mengren Li
Lei Tong
Qiuping Zheng
Yuxiang Yang
Peroxyacetyl nitrate (PAN), a key indicator of photochemical pollution, is generated similarly to ozone (O3), through reactions involving specific volatile organic compounds (VOCs) and nitrogen oxides. Notably, PAN has been observed at unexpectedly high concentrations (maximum: 3.04 ppb) during the summertime. The average daily values of PAN show a strong correlation with black carbon (BC) (R = 0.77) and O3 (R = 0.77), suggesting a close connection between summertime haze and photochemical pollution. We addressed the puzzle of summertime PAN formation and its association with aerosol pollution under high-O3 conditions in Xiamen, a coastal city in southeastern China, by analyzing continuous high-temporal-resolution data utilizing box modeling in conjunction with the Master Chemical Mechanism (MCM) model. The MCM model, with an index of agreement (IOA) value of 0.75, effectively investigates PAN formation, performing better during the clean period (R2: 0.68; slope K: 0.91) than the haze one (R2: 0.47; slope K: 0.75). Using eXtreme Gradient Boosting (XGBoost), we identified NH3, NO, and PM2.5 as the primary factors for simulation bias. Moreover, the net production rate of PAN becomes negative with PAN constrained, suggesting an unknown compensatory mechanism. Both relative incremental reactivity (RIR) and empirical kinetic modeling approach (EKMA) analyses indicate that PAN formation is VOC-controlled. Controlling emissions of VOCs, particularly alkenes, C5H8, and aromatics, would mitigate PAN pollution. PAN promotes OH and HO2 while inhibiting the formation of O3, RO2, NO, and NO2. This study deepens our comprehension of PAN photochemistry while also offering scientific insights for guiding future PAN pollution control strategies.
- Article
(4109 KB) - Full-text XML
-
Supplement
(3493 KB) - BibTeX
- EndNote
PAN is a significant secondary gaseous pollutant commonly present in photochemical smog and poses risk to human health and plant growth, being 1–2 orders of magnitude more phytotoxic than O3 (Yukihiro et al., 2012; Taylor, 1969). Additionally, PAN's low aqueous solubility, minimal reactivity with hydroxyl radicals (OH), and slow photolysis contribute to its capacity for long-range transport of nitrogen oxides (NOx) (Xu et al., 2018; Zhai et al., 2024; Marley et al., 2007). Therefore, its formation in polluted areas holds great importance beyond local concerns. Similarly to surface O3, PAN is produced during the oxidation of volatile organic compounds (VOCs) in the presence of NOx (Reactions R1–R3) (Xu et al., 2021). PAN is formed when NO2 reacts with peroxyacetyl (PA) radicals (CH3C(O)OO•) (Reaction R2), but the presence of NO consumes PA radicals, inhibiting PAN production (Reaction R3), which creates a comparable dependence of PAN and O3 on NO and NO2 levels. Unlike O3, however, PAN is influenced by only a limited number of oxygenated VOCs (OVOCs) that generate PA radicals. These OVOCs, which are second-generation precursors of PAN, include acetaldehyde (CH3CHO), acetone (CH3C(O)CH3), methylglyoxal (MGLY; CH3C(O)CHO), methyl vinyl ketone (MVK; CH2CHC(O)CH3), methyl ethyl ketone (MEK; CH3C(O)CH2CH3), methacrolein (MACR; CH2C(CH3)CHO), and biacetyl (CH3C(O)C(O)CH3). These compounds are typically formed from the oxidation of alkenes, aromatics, and isoprene, which are the first-generation precursors of PAN (Xue et al., 2014; Zhang et al., 2015). Identifying the dominant precursors is crucial for managing PAN pollution effectively. In the troposphere, thermal decomposition (Reaction R4) is the primary process responsible for PAN loss.
In recent years, wintertime photochemical air pollution has increasingly garnered attention. At this time, the concentration of O3 is low due to the strong titration of NO, while the concentration of aerosol is high, and it is found that aerosol promotes PAN generation (Xu et al., 2021). Surprisingly high concentrations of OH radicals, particularly under hazy conditions, have been observed and are largely attributed to HONO photolysis (Xu et al., 2021). Winter photochemical and haze pollution often exacerbate each other, with photochemical trace gases supplying both oxidants and precursors for aerosol formation and aerosols acting as mediums for heterogeneous reactions that produce key oxidants such as HONO, H2O2, and OH radicals (Xu et al., 2021). The OH produced by HONO photolysis can partially replace the UV action to promote PAN formation in winter in the southeast coastal area of China when particulate matter is high (≥ 35 µg m−3) (Hu et al., 2020). Zhang et al. (2020) found the potential HONO sources significantly improved the PAN simulations in wintertime heavy haze events with high concentrations of PAN. High concentrations of PAN are a consequence of the increased levels of precursors and HONO observed during haze episodes (Liu et al., 2018). In conclusion, most previous studies have studied the effect of aerosol on PAN generation in winter. Further research on PAN should determine whether particulates significantly contribute to its formation during warmer seasons with elevated O3 concentrations (Xu et al., 2021). In eastern China, photochemical air pollution often involves high concentrations of both O3 and PAN, a persistent issue during the warm season (April–September) for many years (Lu et al., 2020). The characteristics and formation pathways of PAN during summer have been increasingly studied in regions such as the North China Plain (NCP), the Yangtze River Delta, the Pearl River Delta, and southwestern China. These studies have generally shown consistent diurnal patterns and strong correlations between PAN and O3, identifying acetaldehyde – primarily derived from the degradation of aromatics and alkenes – as the key direct precursor of PAN in the summer. However, there has been limited research on the formation of PAN and its relationship with aerosol pollution during the summertime.
Xiamen is one of the fastest-urbanizing regions in southeast China and is also one of the cities with the best air quality in China, with the air quality possibly representing the future of other Chinese urban regions. Between 2018 and 2023, Xiamen's air quality ranked among the top 10 cities in China, achieving positions of 7th in 2018, 4th in both 2019 and 2020, 6th in 2021, and 9th in 2022, returning to 7th in 2023 (https://www.mee.gov.cn/, last access: 30 October 2024). Xiamen is located in a low-latitude coastal area, with abundant sunlight and long daylight hours during the summer, resulting in strong solar radiation and rapid photochemical conversion rates. The city is typically influenced by the East Asian monsoon and serves as a transport channel for atmospheric pollutants from both the Yangtze River Delta and the Pearl River Delta regions. Additionally, during the summer, Xiamen is often affected by complex meteorological conditions such as typhoons and the western Pacific subtropical high (WPSH). The WPSH creates weather conditions that promote the formation and accumulation of photochemical pollutants and particulate matter (Wu et al., 2019). This setting provides an ideal “laboratory” for investigating the complexities of summertime PAN formation and its relationship with aerosol pollution under high O3 concentrations. In summer, especially in July, high temperatures, high humidity, and intense radiation are likely to accelerate both the formation and the consumption rates of PAN. In this study, continuous measurements of trace gases, substances related to aerosols, photolysis rate constants, and meteorological parameters were performed at a suburban site in Xiamen from 10 to 31 July 2018. Firstly, we provide an overview of pollutant concentrations, meteorological parameters, and weather conditions during the observation period. Secondly, we simulate PAN concentration with the aid of box modeling combined with the Master Chemical Mechanism (MCM) model. Using machine learning with eXtreme Gradient Boosting (XGBoost), we identified the key factors that affect the observation-based model (OBM) simulation results and clarified the mechanisms linking haze pollution to photochemical air pollution, as indicated by PAN and O3. Thirdly, the study identifies the main precursors and oxidants responsible for summertime PAN production in Xiamen and evaluates the influence of PAN on local atmospheric oxidation capacity. This study further emphasizes the interplay between haze and photochemical air pollution and highlights significant implications for future research.
2.1 Field observations
Trace gases (including PAN, O3, HONO, HNO3, HCl, NH3, VOCs, NOx, CO, and SO2), substances related to aerosols (including BC, PM1, PM2.5, PM10, SO, NO, NH, Cl−, Na+, K+, Ca2+, and Mg2+), photolysis rate constants (including JO1D, JNO2, JHONO, JHCHO_M, JHCHO_R, JNO3_M, JNO3_R, and JH2O2), and meteorological parameters (including temperature, relative humidity, atmospheric pressure, wind speed, and wind direction) were continuously measured at a suburban site in Xiamen from 10 to 31 July 2018. All instruments were placed inside an air-conditioned container situated on the rooftop of a 20-story building at the Institute of Urban Environment (IUE), Chinese Academy of Sciences (24.61° N, 118.06° E) (Fig. S1a in the Supplement). When southerly winds prevailed, Xiamen Island, characterized by a dense population and traffic congestion, was located upwind of IUE (Fig. S1b). The IUE supersite is surrounded by Xinglin Bay; several universities and institutes; and major roadways with heavy traffic, such as Jimei Road (< 200 m away), the Shenhai Expressway (870 m away), and the Xiasha Expressway (2300 m away) (Fig. S1c).
PAN measurements were conducted using a PANs-1000 analyzer (Focused Photonics Inc., Hangzhou, China), which features an automated system that consists of a gas chromatograph, an electron capture detector, and a calibration unit. The analyzer provided PAN readings every 5 min, with a detection limit of 50 ppt. The uncertainty and precision of the PAN measurements were ±10 % and 3 %, respectively. The PAN standard gas was produced through the reaction of acetone and NO under UV light. Calibration procedures included monthly multi-point calibrations and weekly single-point calibrations. Detailed information about the PAN detection system and calibration can be found in previous studies (Hu et al., 2020; Liu et al., 2022a). The VOC measurements were conducted using a gas chromatography mass spectrometer (GC-FID/MS, TH-300B, Wuhan, China) at an hourly time resolution. Detailed information regarding the VOC detection system and calibration procedures is available in our previous study (Liu et al., 2022b). HONO measurements were conducted using a customized incoherent broadband cavity-enhanced absorption spectroscopy (IBBCEAS) system developed by the Anhui Institute of Optics and Fine Mechanics (AIOFM), Chinese Academy of Sciences. The HONO detection limit was 100 ppt, with a time resolution of 1 min. The measurement principle and calibration method of IBBCEAS can be found in the previous literature (Hu et al., 2022; Duan et al., 2018; Hu et al., 2024). The concentrations of inorganic components in PM2.5 aerosols (including SO, NO, NH, Cl−, Na+, K+, Ca2+, Mg2+), as well as the concentrations of gases such as NH3, HCl, and HNO3, were analyzed using a Monitor for AeRosols and Gases in ambient Air (MARGA; model ADI 2080, Applikon Analytical B.V., the Netherlands) (Hu et al., 2022). The criteria air pollutants O3, NOx, CO, and SO2 were measured using different methods: ultraviolet (UV) absorption for O3 (TEI model 49i), chemiluminescence with a molybdenum converter for NOx (TEI model 42i), non-dispersive infrared for CO (TEI model 48i), and pulsed UV fluorescence for SO2 (TEI model 43i). A tapered element oscillating microbalance (TEOM 1405, Thermo Fisher Scientific, MA, USA) was used to continuously measure the mass concentrations of PM1, PM2.5, and PM10 online. A photolysis spectrometer (PFS-100, Focused Photonics Inc., Hangzhou, China) was employed to measure the photolysis rate constants. An ultrasonic atmospherium (150WX, Airmar, USA) was used to measure meteorological parameters.
2.2 Box modeling
This study employed a box model framework utilizing the Master Chemical Mechanism (MCMv3.3.1, https://mcm.york.ac.uk/MCM/home.htt, last access: 30 July 2024) to investigate sensitivity and mechanisms of PAN formation. The model constraints were derived from observations of trace gases and meteorological parameters, which were averaged to 1 h intervals. The reliability of model simulation results is often assessed using the index of agreement (IOA), which ranges from 0 to 1, with a higher IOA signifying greater alignment between observed and simulated values. Note that the model simulation values at this time are not constrained by PAN. For specific formulas, please refer to the Supplement (Eq. S1). Other formulas, including PAN production rates (P(PAN)), the net production of PAN (Net(PAN)), and the relative incremental reactivity (RIR), are provided in the Supplement (Eqs. S2–S4).
The MCM simulates the nonlinear interaction between PAN and its precursors by altering the VOC-to-NOx ratio across multiple scenarios while keeping all other parameters fixed. In this study, a 20 % step size was applied, reducing VOCs and NOx from 200 % down to 0 % to construct a scenario matrix. A total of 121 scenarios were generated to model the PAN production rate. The scenario representing the average VOCs and NOx mixing ratio during the sampling period was designated as the base case, with the remaining 120 scenarios created by systematically adjusting the VOC-to-NOx ratio. The output from these 121 simulations was used to construct isopleth diagrams depicting the relationship between VOCs, NOx, and PAN.
2.3 Machine learning model
To identify the key factors influencing the performance of the model simulation, the machine learning (ML) model was applied to establish the prediction model of bias between simulation by OBM and observation. XGBoost is a supervised boosting algorithm that reduces the risk of over-fitting, captures the nonlinear relationships among predictor variables, and solves numerous data science problems in a rapid and accurate way (Li et al., 2024). It has demonstrated high performance in O3 studies in China. As compared to other bagging tree models like random forest, XGBoost can handle more complex data while consuming fewer computing resources. To further improve the interpretability of the ML model, the feature importance of independent input variables in the XGBoost model is quantified using the SHapley Additive exPlanations (SHAP) approach (Lin et al., 2024). The SHAP approach calculates a value that represents the contribution of each feature to the model's outcome, which has been successfully applied in atmospheric environmental studies (Li et al., 2024; Lin et al., 2024). When the model was being adjusted, 90 % of the data were used as the training data set and 10 % of the data were used as the test data set. The hyperparameters were tuned using grid search and a cross-validation method. Specifically, for a single hyperparameter, a grid search was used to obtain its more appropriate value range, and for the combinations of hyperparameters, the whole training set was split into 10 folds and then a grid search was run over pre-adjusted combinations of hyperparameters by training 9 folds and predicting on the remaining 1 fold in a cross-validation procedure. For key hyperparameters of the XGBoost model, the number of trees was 100, the learning rate was 0.1, and the max depth was 6. The model was trained and tested on hourly data during the whole observation, and the established model was examined by the coefficient of determination (R2) value, the root-mean-square error (RMSE), and mean absolute error (MAE). The formulas of RMSE and MAE are provided in the Supplement (Eqs. S5 and S6). The performance of both models is illustrated in Fig. S3. The R2, MAE, and RMSE for the training set are 0.90, 0.08, and 0.12, respectively, while the corresponding values for the test set are 0.77, 0.10, and 0.14, respectively. These statistical metrics indicate that the XGBoost model is promising for further analysis.
3.1 Overview of observation
The measured data of PAN, related trace gases, and meteorological parameters at IUE over 10 to 31 July 2018 are documented in Fig. 1. Combined with the synoptic situation shown in Fig. S4, the eighth typhoon of 2018, Typhoon Maria, made landfall on the morning of 11 July at Huangqi Peninsula in Lianjiang County, Fujian. Due to the influence of the typhoon's outer-spiral rain bands, there was moderate to heavy rain on 11 July 2018. Correspondingly, there was a noticeable decrease in ultraviolet radiation and the temperatures. Starting from 12 July 2018, a WPSH strengthened and extended westward, exerting control over Xiamen. In the lower atmosphere, it was influenced by the eastward flow, resulting in predominantly cloudy weather. From 16 to 18 July 2018, the area was affected by the outer periphery of Typhoon Shanshen, which formed on 17 July 2018, in the northeastern part of the South China Sea and moved westward, making landfall along the coast of the town of Wancheng in the city of Wanning, Hainan Province, in the early hours of 17 July 2018. During this period, the city experienced strong winds with gusts reaching 5 to 6 on the Beaufort Scale in the urban areas. At the same time, the concentration of various pollutants reached their lowest levels, and the daily variation patterns were less pronounced. From 20 to 21 July 2018, Xiamen experienced the influence of the peripheral descending airflow associated with Typhoon Ampil (which formed in the northwest Pacific Ocean around 20:00 local time (LT) on 18 July 2018 and moved northwest, making landfall along the coast of Chongming Island, Shanghai, around noon on 22 July 2018). During this period, there were fewer clouds and higher temperatures. From 22 to 24 July 2018, the city was successively affected by the outer periphery of Typhoon Ampil and a tropical low-pressure system, resulting in occasional showers or thunderstorms. From 25 to 31 July 2018, a WPSH once again strengthened and controlled Xiamen. As a result, Xiamen experienced stable meteorological conditions, with light winds (wind speed (WS) = 1.04 m s−1), persistently high temperatures (maximum daily average of 37.82 °C), and high relative humidity (maximum daily average of 81.65 %). These factors created an environment that favored the buildup of particulate matter and enhanced the photochemical formation of O3 and PAN (Wu et al., 2019). The maximum daily averages of PM2.5, O3, and PAN were 49.26 µg m−3, 93.62 ppb, and 1.37 ppb, respectively.
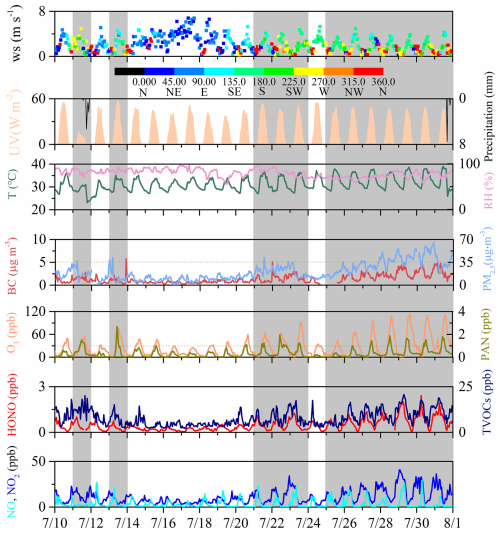
Figure 1Time series of trace gases and meteorological parameters observed at IUE during 10–31 July 2018 (here and similarly in other figures, the date format is month/day). The gray shading represents days when the PM2.5 hourly daily maximum value exceeded 35 µg m−3.
The phenomenon of simultaneous high levels of photochemical and particulate matter appears. Throughout the 22 d campaign, 12 dates (including 11, 13, 21–23, and 25–31 July 2018) were observed with 1 h concentrations of PM2.5 exceeding 35 µg m−3 and 13 dates (including 11, 13, 15, 20–23, and 26–31 July 2018) were observed with 5 min concentrations of PAN exceeding 1 ppb. The maximum concentration was recorded at 3.04 ppb (5 min data) at 11:09 LT on 13 July 2018. This concentration of PAN is comparable to the levels recorded downwind of Guangzhou, southern China (3.9 ppb; Wang et al., 2010), and to 2.51 ppb in Nashville, TN, USA (Roberts et al., 2002). However, this value was significantly lower than heavily polluted areas in northern China in the summer, such as Beijing (9.34 ppb; Xue et al., 2014), Lanzhou (9.12 ppb; Zhang et al., 2009), and Jinan (13.47 ppb; Liu et al., 2018). This is likely because the higher summer temperatures in the southeastern coastal region are conducive to the thermal decomposition of PAN, and the precursor concentrations of PAN, including NO2 and VOCs, are significantly lower in the studied area compared to those in the northern region. The concentration of alkanes is the highest, followed by alkenes, OVOCs, and aromatics, while halogenated hydrocarbons and C5H8 exhibit lower concentrations (Fig. S5). Furthermore, VOC concentrations for various species are elevated during haze periods compared to clean periods (Fig. S5). Table S1 in the Supplement provides the detailed VOC concentrations observed during the study period. Throughout the observation period, the variations in O3 and PAN were almost identical, but the maximum concentration of O3 occurred at 15:00 LT on 29 July 2018 (114.12 ppb). The correlation between the average daily values of PAN and both BC and O3 is strong, with a correlation coefficient of 0.77 for each (Fig. S6), suggesting that summertime haze and photochemical pollution were deeply connected.
We use the categories “haze” and “clean” based on whether the PM2.5 hourly daily maximum value is greater than 35 µg m−3. Specifically, haze includes 11, 13, 21–23, and 25–31 July 2018, while other days are categorized as clean. To provide a quantitative perspective, the statistics for PAN and associated species were calculated and are compiled in Table 1. PM2.5 concentrations during the haze period were significantly higher than those during the clean period, being 2.49 times those of the clean period. There was no significant difference in UV levels between clean and haze periods, while temperatures in the haze phase were notably higher than those in the clean phase. Therefore, without considering precursors, PAN concentrations should be lower during the haze phase due to higher thermal decomposition. In fact, PAN concentrations during the haze period were 2.35 times higher than those during the clean period. During the haze period, O3 concentrations were also significantly higher than those during the clean period, being 2.04 times those of the clean period. These observations indicate that the atmospheric oxidation capacity is relatively strong during the haze period. Similarly to PAN, HONO also exhibits higher concentrations during the haze phase (approximately 2.33 times the concentration of clean conditions), which is consistent with current research findings that particles promote the generation of HONO (Ye et al., 2017). NO also experienced an increase from clean (3.28 ppb) to hazy (4.30 ppb) conditions, albeit less prominently than NO2 (from 7.21 to 14.55 ppb). This observation further underscores that, during hazy periods, the atmosphere demonstrates heightened oxidizing potential, facilitating the conversion of NO to NO2. While the increased NO levels on hazy days reduced PA radicals and hindered PAN formation, this effect was offset by the concurrently higher concentrations of PAN precursors (NO2 and VOCs) during those days. The total VOCs (TVOCs) have increased to some extent, but in hazy conditions, they are only 1.34 times those of clean conditions. This is also because the strongly oxidizing conditions during haze periods convert VOCs into secondary pollutants, such as O3 and PAN. Although it is acknowledged that VOCs can also be converted into secondary organic aerosol (SOA), the discussion of SOA is beyond the scope of this study. The TVOC levels at this site are comparable to those at a rural site in a coastal city – Qingdao (7.6 ppb); significantly lower than inland sites, such as Wuhan (30.2 ppb; X. Liu et al., 2021) and Chengdu (28.0 ppb; Yang et al., 2020), or economically more developed coastal cities, such as Shanghai (25.3 ppb; Zhu et al., 2020) and Hong Kong SAR (26.9 ppb; Wang et al., 2018); and significantly higher than regional background locations like the Wuyi Mountains (4.7 ppb; Hong et al., 2019) and the Nanling Mountains (4.7 ppb; Wang et al., 2023) and the global background station at Mt. Waliguan (2.6 ppb; Xue et al., 2013). The isoprene level during the haze period was significantly higher than that during the clean period, probably due to the haze period with higher temperature (Wang et al., 2023). The wind speed was very low during both the clean and the haze periods, especially during the haze period, with only 1.12 m s−1. The relative humidity was high during both periods, and there was no significant difference between the clean and haze periods.
Table 1Descriptive statistics of major trace gases (ppb), particulate matter (µg m−3), and meteorological parameters during 10–31 July 2018.

*, , and indicate that the values passed a significance test at 0.05, 0.01, and 0.001 levels, respectively.
The average diurnal patterns of PAN and related variables have been averaged separately for clean and hazy conditions (Fig. 2). The daily variation in PAN exhibits a clear unimodal pattern, with concentrations starting to rise after sunrise and decreasing after 12:00 LT, caused by thermal decomposition of PAN at high temperatures (Fig. 2). Although PAN and O3 exhibit a slight bimodal pattern during the clean period, this is primarily due to the bimodal pattern of UV during this time. The peak occurring at noon indicates that PAN primarily originates locally, as a delay of about 1–2 h would be expected if it were influenced by transportation (Liu et al., 2024). The daytime increment was much larger for hazy conditions (1.17 ± 0.44 ppb) than for clean conditions (0.52 ± 0.21 ppb), indicating stronger photochemical production of PAN for hazy conditions. The daily variation pattern of O3 is similar to that of PAN, except that O3 reaches its peak relatively late compared to PAN, with the peak occurring at 16:00 LT during the clean phase and 14:00 LT during the haze phase. Although PAN and O3 are both products of photochemical reactions involving NOx and VOCs, their production efficiencies differ. PAN is specifically formed from VOCs that are precursors to the acetyl radical (CH3CO), whereas O3 can be produced from the oxidation of any VOCs. Analyzing the correlation between PAN and O3 can offer insights into their respective photochemical production efficiencies. As shown in Fig. S7, the positive correlation between the daily maximum values of PAN and O3 for clean conditions (R2 = 0.67) was better than that for hazy conditions (R2 = 0.15). The slopes of the linear regression were 0.021 ppb ppb−1 for clean conditions and 0.009 ppb ppb−1 for hazy conditions. This indicates that, on average, approximately 2.1 ppb of PAN could be produced for every 100 ppb of O3 formed under clean conditions and about 0.9 ppb of PAN could be produced for every 100 ppb of O3 under hazy conditions in the air masses reaching IUE. The slope of linear regression for clean condition is comparable to those determined in Hong Kong SAR (0.028, Xu et al., 2015), Mexico City (0.020, Marley et al., 2007), and Nashville (0.025, Roberts et al., 2002). The lower efficiency of PAN production relative to O3 indicates that PAN precursors represent only a small portion of the total VOCs, especially during hazy conditions. Additionally, the high temperatures in the southeast coastal region likely contribute to the lower production efficiency of PAN. The average temperature during the entire observation period was 31.39 °C, with an average temperature of 34.64 °C at 12:00 LT. This result is consistent with the result that RIR during the cleaning period is higher than that during the haze period. As shown in Fig. S8, in the clean period, the correlation between PAN and O3 is the strongest (R2 = 0.70), indicating that O3 and PAN are both photochemical end products during clean periods. In contrast, during hazy periods, the correlation between PAN and O3 × JO1D is the strongest (R2 = 0.66), suggesting that O3 plays a more significant role in promoting PAN formation through photolysis to generate OH during hazy periods.

Figure 2The diurnal variations in PAN, HONO, O3, and UV during clean (a) and hazy (b) periods; the diurnal variations in PM2.5, NO, and NO2 during clean (c) and hazy (d) periods; and the diurnal variations in isoprene (C5H8), alkenes, halogenated hydrocarbons (Halo), OVOCs, aromatics (Arom), and alkanes during clean (e) and hazy periods (f).
Unlike the daily variation patterns of PAN and O3, HONO exhibits a swift concentration decrease after sunrise in both clean and hazy conditions, undergoing photolytic conversion into OH radicals. Subsequently, in clean conditions, HONO starts to increase in concentration after sunset. In hazy conditions, however, the increase begins from 16:00 LT and not after sunrise. This suggests a robust daytime net production or transport of HONO, where the rates surpass those of HONO photolysis and other sinks in the afternoon in hazy conditions. The NO levels reach their peak at 07:00 LT during the morning rush hour, reflecting advection of fresh urban plumes to the study site. The daily variation in NO2 exhibits a U shape, reaching its minimum value at 13:00 LT, mainly owing to the effects of emissions, boundary layer height, and photochemical reactions. In the clean period, the daily variation in PM2.5 is similar to that of NO2, both showing a U shape and reaching their lowest values at noon. However, during the haze phase, the daily variation pattern of PM2.5 appears somewhat different. There is a noticeable trough in the early morning, levels remain stable during the day, and they start to rise after sunset. The diurnal variation in VOC concentrations for various species is not significant during clean periods (Fig. 2e), likely due to higher wind speeds that facilitate the dispersion of pollutants. In contrast, during haze periods, the daily variations are evident, with peaks occurring before sunrise, followed by a decline and then an increase after sunset (Fig. 2f). This is because the haze period is relatively stable at nighttime, which allows for the accumulation of pollutants, while during the daytime, sunlight converts VOCs into photochemical products like O3 and PAN.
3.2 PAN formation: key factors and mechanisms
To investigate the key factors and mechanisms of PAN formation, PAN was simulated by constraining the MCM-based box model with meteorological conditions and observed concentrations of precursor gases. The model successfully replicated the variations in PAN, achieving an IOA of 0.75 (Fig. 3a), which was within the accepted range (0.66–0.87) in previous studies (Zeng et al., 2019). The model captured its formation rate well in general, with observed rates varying from 0.04 to 0.52 ppb h−1 (average: 0.20 ppb h−1) and modeled rates ranging from 0.09 to 0.46 ppb h−1 (average: 0.19 ppb h−1) (see Fig. 3b). A similar result was found in the North China Plain (NCP) region in the wintertime (Xu et al., 2021). When calculating the IOA separately for clean and hazy periods, it was found that the IOA significantly increased to 0.89 and 0.81 (Fig. 3c), respectively. This phenomenon indicates a substantial difference in the PAN production and destruction mechanisms between clean and hazy periods. Furthermore, the simulated values are closer to the observed values during the clean period, reflected in a higher R2 value (R2 = 0.68) and a slope value (K) closer to 1 (K = 0.91) (Fig. 3c). In contrast, the R2 value and the K value during the hazy period are only 0.47 and 0.75, respectively (Fig. 3c). This phenomenon suggests that some reactions related to PAN generation or destruction might be missing in the MCM during the hazy period.

Figure 3Comparisons of observed (obs) PAN and simulated (sim) PAN (daytime photochemical PAN production periods indicated by cyan shading) and (b) production rates, as well as (c) correlation between PAN observations and simulated values.
To identify the key factors influencing the performance of the OBM simulation, the bias (the model simulation minus the observed value) was the target. The remaining variables, which were not input into OBM, such as NH3, HNO3, HCl (alkaline and acidic gaseous pollutants), and PM2.5 concentrations and their components, as well as physical process parameters like wind speed and wind direction, were used as features. As shown in Fig. 4a, through XGBoost–SHAP machine learning, we found that NH3 is the most significant parameter affecting bias, contributing 19.68 % (Fig. S9). A scatterplot analysis of the SHAP values of NH3 versus NH3 concentrations revealed that as NH3 concentrations increase (Fig. 4b), OBM tends to overestimate PAN concentration more significantly. To date, there are very few studies that directly address the impact of NH3 on PAN formation. Xu et al. (2021) suggested that NH3 could promote the formation of HONO, which in turn affects PAN formation. However, since we included HONO as an input to constrain the model, the indirect influence of NH3 on PAN formation through HONO can be excluded. NH3 in the atmosphere can preferentially react with sulfuric acid (H2SO4) to form ammonium sulfate ((NH4)2SO4) secondary inorganic aerosols (Behera et al., 2013), leading to the heterogeneous reaction removal of PAN by secondary inorganic aerosols (Pratap et al., 2021). This result is validated by the positive correlation between the SHAP values of NH and SO and their respective concentrations (Fig. S10). NO is the second most significant parameter influencing the bias between the two, contributing 11.33 % (Fig. S9). NO has a negative correlation with the bias (Fig. 4c), indicating that higher NO levels lead to more significant underestimation by the model. Considering the significant positive correlation between PAN and NO at the 0.01 level, with a correlation coefficient of 0.37, and the fact that both reach their peaks around noon (Fig. S11), it is likely that they have a common source. PM2.5 is the third most significant parameter (Fig. 4a), contributing 9.40 % (Fig. S9). PM2.5 has a positive correlation with the bias (Fig. 4d), indicating that higher PM2.5 levels lead to more significant overestimation by the model, suggesting that PAN can undergo heterogeneous removal on the surface of PM2.5 in the actual atmosphere (Sun et al., 2022).

Figure 4Feature importance was obtained by the XGBoost–SHAP method (a). The scatterplots between concentration of the three most important features and their SHAP values (b, c, d), colored with the bias (the model simulation minus the observed value).
Figure 5a and b show the average production and destruction rates of PAN during clean and haze periods, as simulated by OBM without PAN constrained. During the haze period, both the production and the destruction rates of PAN are significantly higher than during the clean period. The higher production rate of PAN during the haze period is due to the higher concentration of PAN precursors, while the higher destruction rate is because both the temperature and the PAN concentration are higher. Regarding the net production rate, this is also higher during the haze period than during the clean period, which corresponds to the previously observed diurnal variation. From 06:00 to 12:00 LT during the haze period, the simulated net production rate of PAN is positive, with an average value of 0.19 ppb h−1. During the clean period, from 06:00 to 12:00 LT, the simulated net production rate of PAN is 0.12 ppb h−1. The observed diurnal variation in PAN shows that from 06:00 to 12:00 LT, the average net production rates during the haze and clean periods are 0.20 ppb h−1 (Fig. 2a) and 0.09 ppb h−1 (Fig. 2b), respectively. The model-simulated net production rate is close to the observed net production rate, further indicating that the model can simulate PAN well and also confirming that PAN in summer mainly comes from local production. The net production rate of PAN during the haze period is similar to the summer results in urban areas of the Pearl River Delta (PRD), i.e., 0.17 ppb h−1, while the net production rate of PAN during the clean period is similar to the summer results in rural areas of the PRD, i.e., 0.12 ppb h−1 (Liu et al., 2024).

Figure 5Average diurnal variation in the OBM-simulated production and destruction and net rates of PAN during clean (a) and haze days (b) without PAN constrained. Average diurnal variation in the OBM-simulated production and destruction and net rates of PAN during clean (c) and haze days (d) with PAN constrained. The time here and similarly in other figures is local time.
Figure 5c and d show the average production and destruction rates of PAN during clean and haze periods, as simulated by OBM with PAN constrained. The net production rate of PAN is approximately zero at night during both clean and haze periods, while there is a significant difference in the net production rate during the day. During the clean period, the daytime net production rate of PAN is greater than zero, with an average value of 0.19 ppb h−1. In contrast, during the haze period, the net production rate of PAN is negative from 00:06 to 13:00 LT, with an average value of −0.47 ppb h−1, and positive from 14:00 to 17:00 LT, with an average value of 0.47 ppb h−1. Previous research has shown that an increase in temperature, an increase in PAN concentration, or a decrease in PAN precursors (including VOCs and NO2) can cause the net production rate of PAN to change from positive to negative (Liu et al., 2024). We conducted a correlation analysis of the net production rate of PAN with temperature, PAN, VOCs, and NO2 concentration and found that the net production rate of PAN had the best correlation with PAN concentration (R2 = 0.13), showing a significant negative correlation (k = −0.53, Fig. S12). Additionally, we also observed that when the net production rate of PAN is negative, the PAN concentration is often very high (Fig. S12). As shown in Fig. 6, we conducted sensitivity experiments by reducing the PAN concentration by 80 %, i.e., 0.2 times the observed value, and found that the simulated net production rate of PAN was positive throughout the observation period. Conversely, when the PAN concentration was increased by 140 %, i.e., 2.4 times the observed value, the simulated net production rate of PAN was found to be almost negative throughout the observation period. Besides, we also conducted sensitivity experiments on temperature and found that when simulating winter temperatures, i.e., 0.4 times the observed value, with a temperature range of 9.25–15.29 °C, the simulated net production rate of PAN was positive throughout the observation period. Similarly, when simulating spring and autumn temperatures, i.e., 0.6 times the observed value, with a temperature range of 13.87–23.39 °C, the simulated net production rate of PAN was also positive throughout the observation period. In conclusion, the simulated net production rate of PAN becomes negative with PAN constrained, further suggesting the existence of an unknown compensatory mechanism.

Figure 6Net PAN production rates simulated by OBM at different PAN concentrations (a) and different temperatures (b).
PAN is formed when the PA radical reacts with NO2. Given the swift equilibrium between Reactions (R2) and (R4) at high temperatures, budget analysis of PA's production and consumption pathways is frequently used to detail the mechanisms behind PAN formation (Sun et al., 2020; Liu et al., 2022a, 2024). Figure 7 illustrates the diurnal patterns of the primary production and loss pathways for the PA radical simulated by OBM across different periods. As shown in Fig. 7, during haze days, the rates of PA production and destruction were twice as high as those on clean days. This indicates that radical cycling and photochemical formation were more efficient during haze days, driven by higher temperatures and a greater abundance of precursors (Zeng et al., 2019). The PA radical production rate from PAN thermal decomposition reached its peak at 15:00 LT (3.22 ppb h−1) and 13:00 LT (10.99 ppb h−1) for clean and haze days, perfectly coinciding with the peak temperature time. In addition, the conversion of PAN into PA radicals through thermal decomposition had high exponential correlations with temperature during both haze days (R2 = 0.95) and clean days (R2 = 0.91) (Fig. S13). Previous laboratory experiments also indicated that the thermal decomposition of PAN is exponentially related to temperature (Cox and Roffey, 1977; Senum et al., 1986; Tuazon et al., 1991). The conversion of PAN into PA radical through thermal decomposition during haze days was significantly higher than that during clean days, which was not only enhanced by higher temperature but also maintained by higher PAN concentration during haze days. The thermal decomposition of PAN into PA radicals during the day (05:00–18:00 LT) accounted for 68.22 % and 45.59 % during haze and clean days, respectively. The pathways that did not account for the transformation between PA and PAN reached their peak around noon (11:00 LT), coinciding with the highest solar radiation and the most intense photochemical reactions, which has been observed in spring and autumn at the same site (Liu et al., 2022a).
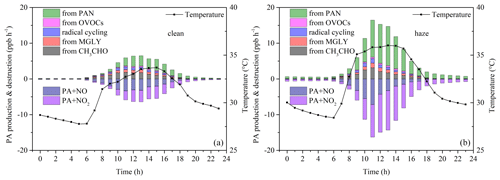
Figure 7PA radical production and destruction pathways simulated by OBM on (a) clean days and (b) haze days.
Production rates of PA from other pathways related to precursors, including OVOCs, radical cycling, MGLY, and CH3CHO, showed single-peak patterns around noon, which suggested that the PA radicals generated from these pathways were primarily increased by intense solar radiation at noontime (Sun et al., 2020). The average daytime PA radical production rates from CH3CHO via reactions with OH and NO3 were 1.10 and 0.93 ppb h−1, accounting for 48.85 % and 49.35 % (exclude PAN thermal decomposition sources) during haze and clean days, respectively. These percentages were comparable to previous studies in Guangzhou (46 %; Yuan et al., 2018), and Beijing (34.11 %–50.19 %; Xue et al., 2014), and a suburban site of Chongqing (47.72 %; Sun et al., 2020). The second production pathway involved MGLY undergoing photolysis and oxidation through reactions with OH and NO3 (haze: 0.50 ppb h−1; clean: 0.42 ppb h−1), contributing to 22.27 % and 22.12 % for haze and clean days, respectively. Subsequently, radical cycling processes – including the decomposition of RO radicals and the reactions of acyl peroxy radicals with NO – were also significant contributors to PA production, accounting for 18.98 % on haze days and 19.54 % on clean days. PA from the other OVOCs (excluding CH3CHO and MGLY) via photolysis and oxidation reactions involving OH, NO3, and O3 accounted for 9.90 % and 8.99 % on haze days (0.22 ppb h−1) and clean days (0.17 ppb h−1). There were no notable differences in the proportions of individual pathways contributing to PA between haze and clean days, indicating comparable pollutant compositions in the atmosphere around IUE (Zeng et al., 2019). In summary, the thermal decomposition of PAN played a dominant role in boosting PA production rates during both clean and haze periods, followed by contributions from CH3CHO, MGLY, radical cycling, and other OVOCs. The primary contributor to the PA destruction rate was the reaction between PA and NO2, accounting for 67.72 % and 51.09 % during haze days (4.74 ppb h−1) and clean days (1.76 ppb h−1), respectively, followed by PA + NO, contributing to 32.28 % and 48.91 % during haze days (2.26 ppb h−1) and clean days (1.69 ppb h−1), respectively.
3.3 Sensitivity of PAN formation and its impact on the local atmosphere
To determine the principal precursors influencing PAN formation, sensitivity modeling analyses were carried out to investigate how PAN relates to its precursors. The RIR reflects how sensitive PAN formation is to changes in its precursor levels. As shown in Fig. 8a, the RIR of NO is negative, ranging from −0.67 to −0.27 (−0.52 ± 0.13) throughout the observation period. However, RIR is positive for other species, with NO2 (0.50 ± 0.11) and VOCs (0.50 ± 0.15) having the highest RIR, followed by HONO (0.12 ± 0.04) and O3 (0.10 ± 0.03). Around 50 types of VOCs were classified as alkanes, OVOCs, halogenated hydrocarbons (Halo), alkenes, aromatics (Arom), and isoprene (C5H8 representing biogenic hydrocarbons). Among these VOCs, the RIR of alkenes (0.22 ± 0.07) is the highest, followed by C5H8 (0.13 ± 0.04) and Arom (0.13 ± 0.04), while OVOCs (0.06 ± 0.01) and Halo (0.05 ± 0.01) have very low RIRs (Fig. 8b). These phenomena indicated that an increased NO level would inhibit the production of PAN, while increased NO2, VOCs (especially alkenes, C5H8, and Arom), HONO, and O3 would promote the production of PAN. Because the values of NO and NO2 RIR are approximately equal but with opposite signs, the RIR for NOx is almost zero, indicating that the PAN generation at this site is not sensitive to NOx. Zeng et al. (2019) also observed that NO2 had a positive effect on PAN formation, while NO had a negative effect in a suburban area of Hong Kong SAR. This finding aligns with the fact that NO2 directly contributes to PAN production, whereas NO reduces PA radicals, thereby inhibiting PAN formation. Based on the scenario analysis (empirical kinetic modeling approach, EKMA), all data points for the 22 d fell above the ridge line (Fig. 8c). A reduction in VOCs at these points resulted in lower PAN concentrations, indicating that PAN formation at IUE was influenced by VOCs and thus was VOC-sensitive. Our previous research also found that in this coastal city, PAN generation is limited by VOCs during the spring and autumn seasons. The difference is that previous studies indicated that reducing NO2, like reducing NO, also leads to an increase in PAN concentration in spring and autumn (Liu et al., 2022a). This is because the NOx concentration in spring and autumn is significantly higher than in summer, which is consistent with both NO2 and NO inhibiting the formation of PAN in regions with high NOx concentrations (Liu et al., 2024).
We divided the RIRs for different species into haze and clean periods and found that the RIRs during clean periods were consistently higher than those during haze periods (Fig. 8d), which indicated that altering the concentrations of these species during clean periods had a greater impact on PAN formation. The rapid thermal decomposition of PAN at high temperatures is likely the primary reason for this. During the haze period, the main source of PA radicals was PAN decomposition, which accounted for 68.22 %, and the other sources were smaller than that during the clean period (the source of the PA radicals will be demonstrated in the following paragraph). Therefore, the sensitivity of PAN production to precursors and HONO and O3 producing OH became lower during the haze period (Y. Liu et al., 2021; T. Liu et al., 2022a).
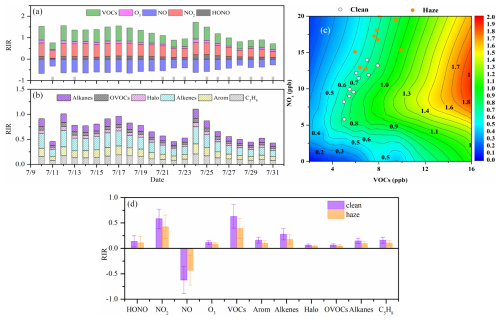
Figure 8These four panels illustrate the RIR of PAN formation to major precursors (a), the impact of different VOC species (b), the isopleth diagrams of PAN formation (c), and a comparison of RIRs between clean and polluted (haze) periods (d).
As shown in Fig. 9, ΔHO2 and ΔOH are positive for most periods, accounting for 72.16 % and 70.83 %, respectively, indicating that the PAN mechanism promotes the generation of HO2 and OH. Over the entire period, ΔHO2 is ppb, with no significant difference between clean and hazy periods, with values of and ppb, respectively (Table S2). OH behaves similarly, with ΔOH being ppb over the entire period, and also shows no significant difference between clean and hazy periods, with values of and ppb, respectively (Table S2). The increase in simulated OH and HO2 concentrations suggests that PAN photochemistry is in favor of radical formation and atmospheric oxidative capacity at this site (Liu et al., 2024). Unlike HO2 and OH, ΔRO2 and ΔNO2 are negative for most periods, accounting for 53.22 % and 67.23 %, respectively, because PAN formation uses up PA and NO2 and the reduction in PA leads to a decrease in the amount of RO2. Over the entire period, ΔRO2 is ppb, with no significant difference between clean and hazy periods, with values of and ppb, respectively (Table S2). The average value of ΔNO2 during the entire observation period is −0.17 ppb, with significant differences between hazy and clean periods (Table S2). Specifically, ΔNO2 is −0.22 ppb during hazy periods and only −0.11 ppb during clean periods, indicating that the PAN mechanism consumes more NO2 during hazy periods. Although ΔNO is positive for most periods, accounting for 78.79 %, the overall mean is −0.01 ppb, with significant differences between hazy and clean periods (Table S2). ΔNO is −0.05 ppb during hazy periods, showing an inhibitory effect, while it is 0.03 ppb during clean periods, showing a promoting effect.
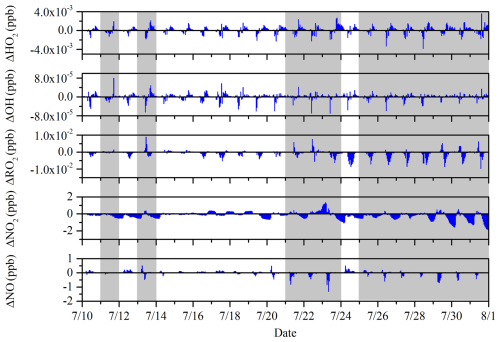
Figure 9The time series of ΔHO2, ΔOH, ΔRO2, ΔNO2, and ΔNO. The ΔHO2, ΔOH, ΔRO2, ΔNO2, and ΔNO are calculated as the base scenario with the PAN mechanism minus the scenario without the PAN mechanism.
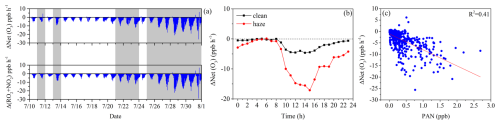
Figure 10(a) Time series plot of ΔNet (O3) and the reaction of Δ(RO2+NO2), (b) diurnal variation in ΔNet(O3) during clean and hazy conditions, and (c) correlation between ΔNet(O3) and PAN. ΔNet(O3) and Δ(RO2 + NO2) are calculated as the base scenario with the PAN mechanism minus the scenario without the PAN mechanism.
As shown in Fig. 10a, the PAN mechanism inhibited 85.80 % of net O3 production during the entire observation period, with inhibition rates (the percentage of negative ΔNet(O3)) of 83.75 % and 87.50 % during clean and haze periods, respectively. This result is consistent with previous spring observations at the same site, where the inhibition rate was 83 % (Liu et al., 2022a). The PAN mechanism mainly inhibits the net O3 generation by increasing the RO2+NO2 reaction (Fig. 10a), with a negligible impact of other reactions (Fig. S14). As shown in Fig. 10b, the diurnal variation trend indicates that the PAN mechanism's inhibitory effect on O3 is significantly greater during haze periods than during clean periods. Additionally, regardless of whether it is during haze periods or clean periods, the PAN mechanism's inhibitory effect on O3 is significantly greater during the day than at night. These phenomena all indicate that the higher the PAN concentration, the more pronounced the inhibitory effect of the PAN mechanism on O3 (Fig. 10c). Under the condition of low precursors (including NOx and VOCs), competition among these precursors may limit their secondary transformation, thus resulting in inhibition (Liu et al., 2024).
This study thoroughly investigated the summertime PAN formation mechanism and established its connection to haze pollution. In addition to NO and TVOCs, the concentration of all pollutants during the haze period is above twice that during the clean period, indicating that the oxidation of NO and TVOCs during the haze period is stronger, which is conducive to the oxidation of NO and TVOCs into secondary pollutants, such as O3 and PAN. The slopes of linear regression between the daily maximum values of PAN and O3 were 0.021 and 0.009 ppb ppb−1 for clean and hazy conditions, respectively, implying that PAN precursors accounted for only a small fraction of the total VOCs, especially in hazy conditions. High temperature should be another factor contributing to the lower production efficiency of PAN in the southeast coastal region. During the whole observation period, the IOA is 0.75, indicating that the MCM model is well suited for exploring the photochemical formation of PAN. During the clean period, simulation results were better than during the haze period (R2: 0.68 vs. 0.47; slope K: 0.91 vs. 0.75), indicating that some reactions related to PAN generation or destruction might be missing in the MCM during the hazy period. Additionally, the simulated net production rate of PAN becomes negative with PAN constrained. However, the observed increase in PAN concentrations indicates that the actual net production rate is positive, suggesting that there are additional sources contributing to PAN generation that are not considered in the MCM. Through XGBoost–SHAP machine learning and given the significant positive correlation between PAN and NO (R = 0.37) at the 0.01 level and their peaks around noon, they likely share a common source. Both RIR and EKMA indicate that PAN formation in this region is VOC-controlled. Controlling emissions of VOCs, particularly alkenes, C5H8, and aromatics, would be beneficial for mitigating PAN pollution. The RIR results also show that during the clean period, PAN is more sensitive to changes in various pollutants than during the haze period, highlighting the great importance of large emission reductions. The PAN mechanism promoted the generation of HO2 and OH, while it inhibited formation of O3, RO2, NO, and NO2. This study improves our thorough understanding of PAN photochemistry and offers valuable scientific guidance for the future management of PAN pollution.
The observation data at this site are available from the authors upon request.
The supplement related to this article is available online at: https://doi.org/10.5194/acp-25-905-2025-supplement.
BH: methodology, formal analysis, investigation, data curation, writing (original draft). NC: software, formal analysis. RL: software, formal analysis. MH: software. JC: funding acquisition, supervision, writing (review and editing). YH: formal analysis. LX: investigation. XF: investigation. ML: investigation. LT: investigation. QZ: investigation. YY: writing (review and editing).
The contact author has declared that none of the authors has any competing interests.
Publisher’s note: Copernicus Publications remains neutral with regard to jurisdictional claims made in the text, published maps, institutional affiliations, or any other geographical representation in this paper. While Copernicus Publications makes every effort to include appropriate place names, the final responsibility lies with the authors.
The authors gratefully acknowledge the financial support of the National Natural Science Foundation of China, the Natural Science Foundation of Fujian Province and Zhangzhou City, the President's Fund of Minnan Normal University, the Xiamen Atmospheric Environment Observation and Research Station of Fujian Province, and the Fujian Key Laboratory of Atmospheric Ozone Pollution Prevention (Institute of Urban Environment, Chinese Academy of Sciences).
This work was supported by the National Natural Science Foundation of China (grant nos. 42305102, U22A20578), the guiding project of seizing the commanding heights of “self-purifying city” (grant no. IUE-CERAE-202402), the Natural Science Foundation of Fujian Province (grant no. 2023J05179), the Natural Science Foundation of Zhangzhou City (grant no. ZZ2023J07), and the President's Fund of Minnan Normal University (grant no. KJ2021009). This study was also funded by the Xiamen Atmospheric Environment Observation and Research Station of Fujian Province and the Fujian Key Laboratory of Atmospheric Ozone Pollution Prevention (Institute of Urban Environment, Chinese Academy of Sciences).
This paper was edited by Farahnaz Khosrawi and reviewed by two anonymous referees.
Behera, S. N., Sharma, M., Aneja, V. P., and Balasubramanian, R.: Ammonia in the atmosphere: a review on emission sources, atmospheric chemistry and deposition on terrestrial bodies, Environ. Sci. Pollut. R., 20, 8092–8131, https://doi.org/10.1007/s11356-013-2051-9, 2013.
Cox, R. A. and Roffey, M. J. : Thermal decomposition of peroxyacetylnitrate in the presence of nitric oxide, Environ. Sci. Technol., 11, 900–906, https://doi.org/10.1021/es60132a010, 1977.
Duan, J., Qin, M., Ouyang, B., Fang, W., Li, X., Lu, K., Tang, K., Liang, S., Meng, F., Hu, Z., Xie, P., Liu, W., and Häsler, R.: Development of an incoherent broadband cavity-enhanced absorption spectrometer for in situ measurements of HONO and NO2, Atmos. Meas. Tech., 11, 4531–4543, https://doi.org/10.5194/amt-11-4531-2018, 2018.
Hong, Z., Li, M., Wang, H., Xu, L., Hong, Y., Chen, J., Chen, J., Zhang, H., Zhang, Y., Wu, X., Hu, B., and Li, M.: Characteristics of atmospheric volatile organic compounds (VOCs) at a mountainous forest site and two urban sites in the southeast of China, Sci. Total Environ., 657, 1491–1500, https://doi.org/10.1016/j.scitotenv.2018.12.132, 2019.
Hu, B., Liu, T., Hong, Y., Xu, L., Li, M., Wu, X., Wang, H., Chen, J., and Chen, J.: Characteristics of peroxyacetyl nitrate (PAN) in a coastal city of southeastern China: Photochemical mechanism and pollution process, Sci. Total Environ., 719, 137493, https://doi.org/10.1016/j.scitotenv.2020.137493, 2020.
Hu, B., Duan, J., Hong, Y., Xu, L., Li, M., Bian, Y., Qin, M., Fang, W., Xie, P., and Chen, J.: Exploration of the atmospheric chemistry of nitrous acid in a coastal city of southeastern China: results from measurements across four seasons, Atmos. Chem. Phys., 22, 371–393, https://doi.org/10.5194/acp-22-371-2022, 2022.
Hu, B., Wang, Y., Chen, J., Chen, N., Hong, Y., Xu, L., Fan, X., Li, M., and Tong, L.: The observation of atmospheric HONO by wet-rotating-denuder ion chromatograph in a coastal city: Performance and influencing factors, Environ. Pollut., 356, 124355, https://doi.org/10.1016/j.envpol.2024.124355, 2024.
Li, H., Yang, Y., Su, H., Wang, H., Wang, P., and Liao, H.: Ozone pollution in China affected by climate change in a carbon neutral future as predicted by a process-based interpretable machine learning method, Geophys. Res. Lett., 51, e2024GL109520, https://doi.org/10.1029/2024GL109520, 2024.
Lin, Z., Xu, L., Yang, C., Chen, G., Ji, X., Li, L., Zhang, K., Hong, Y., Li, M., Fan, X., Hu, B., Zhang, F., and Chen, J.: Trends of peroxyacetyl nitrate and its impact on ozone over 2018–2022 in urban atmosphere, npj Climate and Atmospheric Science, 7, 192, https://doi.org/10.1038/s41612-024-00746-7, 2024.
Liu, L., Wang, X., Chen, J., Xue, L., Wang, W., Wen, L., Li, D., and Chen, T.: Understanding unusually high levels of peroxyacetyl nitrate (PAN) in winter in Urban Jinan, China, J. Environ. Sci., 71, 249–260, https://doi.org/10.1016/j.jes.2018.05.015, 2018.
Liu, T., Chen, G., Chen, J., Xu, L., Li, M., Hong, Y., Chen, Y., Ji, X., Yang, C., Chen, Y., Huang, W., Huang, Q., and Wang, H.: Seasonal characteristics of atmospheric peroxyacetyl nitrate (PAN) in a coastal city of Southeast China: Explanatory factors and photochemical effects, Atmos. Chem. Phys., 22, 4339–4353, https://doi.org/10.5194/acp-22-4339-2022, 2022a.
Liu, T., Hong, Y., Li, M., Xu, L., Chen, J., Bian, Y., Yang, C., Dan, Y., Zhang, Y., Xue, L., Zhao, M., Huang, Z., and Wang, H.: Atmospheric oxidation capacity and ozone pollution mechanism in a coastal city of southeastern China: analysis of a typical photochemical episode by an observation-based model, Atmos. Chem. Phys., 22, 2173–2190, https://doi.org/10.5194/acp-22-2173-2022, 2022b.
Liu, T., Wang, Y., Cai, H., Wang, H., Zhang, C., Chen, J., Dai, Y., Zhao, W., Li, J., Gong, D., Chen, D., Zhai, Y., Zhou, Y., Liao, T., and Wang, B.: Complexities of peroxyacetyl nitrate photochemistry and its control strategies in contrasting environments in the Pearl River Delta region, npj Climate and Atmospheric Science, 7, 116, https://doi.org/10.1038/s41612-024-00669-3, 2024.
Liu, X., Guo, H., Zeng, L., Lyu, X., Wang, Y., Zeren, Y., Yang, J., Zhang, L., Zhao, S., Li, J., and Zhang, G.: Photochemical ozone pollution in five Chinese megacities in summer 2018, Sci. Total Environ., 801, 149603, https://doi.org/10.1016/j.scitotenv.2021.149603, 2021a.
Liu, Y., Shen, H., Mu, J., Li, H., Chen, T., Yang, J., Jiang, Y., Zhu, Y., Meng, H., Dong, C., Wang, W., and Xue, L.: Formation of peroxyacetyl nitrate (PAN) and its impact on ozone production in the coastal atmosphere of Qingdao, North China, Sci. Total Environ., 778, 146265, https://doi.org/10.1016/j.scitotenv.2021.146265, 2021b.
Lu, X., Zhang, L., Wang, X., Gao, M., Li, K., Zhang, Y., Yue, X., and Zhang, Y.: Rapid Increases in Warm-Season Surface Ozone and Resulting Health Impact in China Since 2013, Environ. Sci. Tech. Let., 7, 240–247, https://doi.org/10.1021/acs.estlett.0c00171, 2020.
Marley, N. A., Gaffney, J. S., Ramos-Villegas, R., and Cárdenas González, B.: Comparison of measurements of peroxyacyl nitrates and primary carbonaceous aerosol concentrations in Mexico City determined in 1997 and 2003, Atmos. Chem. Phys., 7, 2277–2285, https://doi.org/10.5194/acp-7-2277-2007, 2007.
Pratap, V., Carlton, A. G., Christiansen, A. E., and Hennigan, C. J.: Partitioning of ambient organic gases to inorganic salt solutions: influence of salt identity, ionic strength, and pH, Geophys. Res. Lett., 48, e2021GL095247, https://doi.org/10.1029/2021GL095247, 2021.
Roberts, J. M., Flocke, F., Stroud, C. A., Hereid, D., Williams, E., Fehsenfeld, F., Brune, W., Martinez, M., and Harder, H.: Ground-based measurements of peroxycarboxylic nitric anhydrides (PANs) during the 1999 Southern Oxidants Study Nashville Intensive, J. Geophys. Res.-Atmos., 107, ACH 1-1–ACH 1-10, https://doi.org/10.1029/2001jd000947, 2002.
Senum, G. I., Fajer, R., and Gaffney, J. S. Fourier transform infrared spectroscopic study of the thermal stability of peroxyacetyl nitrate, J. Phys. Chem., 90, 152–156, https://doi.org/10.1021/j100273a034, 1986.
Sun, M., Cui, J. N., Zhao, X., and Zhang, J.: Impacts of precursors on peroxyacetyl nitrate (PAN) and relative formation of PAN to ozone in a southwestern megacity of China, Atmos. Environ., 231, 117542, https://doi.org/10.1016/j.atmosenv.2020.117542, 2020.
Sun, M., Zhou, Y., Wang, Y., Qiao, X., Wang, J., and Zhang, J.: Heterogeneous reaction of peroxyacetyl nitrate on real-world PM2.5 aerosols: Kinetics, influencing Factors, and atmospheric implications, Environ. Sci. Technol., 56, 9325–9334, https://doi.org/10.1021/acs.est.2c03050, 2022.
Taylor, O. C.: Importance of peroxyacetyl nitrate (PAN) as a phytotoxic air pollutant, Journal of the Air Pollution Control Association, 19, 347–351, https://doi.org/10.1080/00022470.1969.10466498, 1969.
Tuazon, E. C., Carter, W. P., and Atkinson, R.: Thermal decomposition of peroxyacetyl nitrate and reactions of acetyl peroxy radicals with nitric oxide and nitrogen dioxide over the temperature range 283-313 K, J. Phys. Chem., 95, 2434–2437, https://doi.org/10.1021/j100159a059, 1991.
Wang, B., Shao, M., Roberts, J. M., Yang, G., Yang, F., Hu, M., Zeng, L., Zhang, Y., and Zhang, J.: Ground-based on-line measurements of peroxyacetyl nitrate (PAN) and peroxypropionyl nitrate (PPN) in the Pearl River Delta, China, Int. J. Environ. An. Ch., 90, 548–559, https://doi.org/10.1080/03067310903194972, 2010.
Wang, H., Lyu, X., Guo, H., Wang, Y., Zou, S., Ling, Z., Wang, X., Jiang, F., Zeren, Y., Pan, W., Huang, X., and Shen, J.: Ozone pollution around a coastal region of South China Sea: interaction between marine and continental air, Atmos. Chem. Phys., 18, 4277–4295, https://doi.org/10.5194/acp-18-4277-2018, 2018.
Wang, Y., Liu, T., Gong, D., Wang, H., Guo, H., Liao, M., Deng, S., Cai, H., and Wang, B.: Anthropogenic pollutants induce changes in peroxyacetyl nitrate formation intensity and pathways in a mountainous background atmosphere in southern China, Environ. Sci. Technol., 57, 6253–6262, https://doi.org/10.1021/acs.est.2c02845, 2023.
Wu, X., Xu, L., Hong, Y., Chen, J., Qiu, Y., Hu, B., Hong, Z., Zhang, Y., Liu, T., Chen, Y., Bian, Y., Zhao, G., Chen, J., and Li, M.: The air pollution governed by subtropical high in a coastal city in Southeast China: Formation processes and influencing mechanisms, Sci. Total Environ., 692, 1135–1145, https://doi.org/10.1016/j.scitotenv.2019.07.341, 2019.
Xu, W., Zhang, G., Wang, Y., Tong, S., Zhang, W., Ma, Z., Lin, W., Kuang, Y., Yin, L., and Xu, X.: Aerosol promotes peroxyacetyl nitrate formation during winter in the North China Plain, Environ. Sci. Technol., 55, 3568–3581, https://doi.org/10.1021/acs.est.0c08157, 2021.
Xu, X., Zhang, H., Lin, W., Wang, Y., Xu, W., and Jia, S.: First simultaneous measurements of peroxyacetyl nitrate (PAN) and ozone at Nam Co in the central Tibetan Plateau: impacts from the PBL evolution and transport processes, Atmos. Chem. Phys., 18, 5199–5217, https://doi.org/10.5194/acp-18-5199-2018, 2018.
Xu, Z., Xue, L., Wang, T., Xia, T., Gao, Y., Louie, P. K. K., and Luk, C. W. Y.: Measurements of peroxyacetyl nitrate at a background site in the Pearl River Delta region: production efficiency and regional transport, Aerosol Air Qual. Res., 15, 833–841, https://doi.org/10.4209/aaqr.2014.11.0275, 2015.
Xue, L., Wang, T., Wang, X., Blake, D. R., Gao, J., Nie, W., Gao, R., Gao, X., Xu, Z., Ding, A., Huang, Y., Lee, S., Chen, Y., Wang, S., Chai, F., Zhang, Q., and Wang, W.: On the use of an explicit chemical mechanism to dissect peroxy acetyl nitrate formation, Environ. Pollut., 195, 39–47, https://doi.org/10.1016/j.envpol.2014.08.005, 2014.
Xue, L. K., Wang, T., Guo, H., Blake, D. R., Tang, J., Zhang, X. C., Saunders, S. M., and Wang, W. X.: Sources and photochemistry of volatile organic compounds in the remote atmosphere of western China: results from the Mt. Waliguan Observatory, Atmos. Chem. Phys., 13, 8551–8567, https://doi.org/10.5194/acp-13-8551-2013, 2013.
Yang, X., Wu, K., Wang, H., Liu, Y., Gu, S., Lu, Y., Zhang, X., Hu, Y., Ou, Y., Wang, S., and Wang, Z.: Summertime ozone pollution in Sichuan Basin, China: Meteorological conditions, sources and process analysis, Atmos. Environ., 226, 117392, https://doi.org/10.1016/j.atmosenv.2020.117392, 2020.
Ye, C., Zhang, N., Gao, H., and Zhou, X.: Photolysis of particulate nitrate as a source of HONO and NOx, Environ. Sci. Technol., 51, 6849–6856, https://doi.org/10.1021/acs.est.7b00387, 2017.
Yuan, J., Ling, Z., Wang, Z., Lu, X., Fan, S., He, Z., Guo, H., Wang, X., and Wang, N.: PAN–precursor relationship and process analysis of PAN variations in the Pearl River Delta region, Atmosphere, 9, 372, https://doi.org/10.3390/atmos9100372, 2018.
Yukihiro, M., Hiramatsu, T., Bouteau, F., Kadono, T., and Kawano, T.: Peroxyacetyl nitrate-induced oxidative and calcium signaling events leading to cell death in ozone-sensitive tobacco cell-line, Plant Signaling & Behavior, 7, 113–120, https://doi.org/10.4161/psb.7.1.18376, 2012.
Zeng, L., Fan, G. J., Lyu, X., Guo, H., Wang, J. L., and Yao, D.: Atmospheric fate of peroxyacetyl nitrate in suburban Hong Kong and its impact on local ozone pollution, Environ. Pollut., 252, 1910–1919, https://doi.org/10.1016/j.envpol.2019.06.004, 2019.
Zhai, S., Jacob, D. J., Franco, B., Clarisse, L., Coheur, P., Shah, V., Bates, K. H., Lin, H., Dang, R., Sulprizio, M. P., Huey, L. G., Moore, F. L., Jaffe, D. A., and Liao, H.: Transpacific transport of Asian peroxyacetyl nitrate (PAN) observed from satellite: implications for ozone, Environ. Sci. Technol., 58, 9760–9769, https://doi.org/10.1021/acs.est.4c01980, 2024.
Zhang, G., Mu, Y., Zhou, L., Zhang, C., Zhang, Y., Liu, J., Fang, S., and Yao, B.: Summertime distributions of peroxyacetyl nitrate (PAN) and peroxypropionyl nitrate (PPN) in Beijing: Understanding the sources and major sink of PAN, Atmos. Environ., 103, 289–296, https://doi.org/10.1016/j.atmosenv.2014.12.035, 2015.
Zhang, J., Guo, Y., Qu, Y., Chen, Y., Yu, R., Xue, C., Yang, R., Zhang, Q., Liu, X., Mu, Y., Wang, J., Ye, C., Zhao, H., Sun, Q., Wang, Z., and An, J.: Effect of potential HONO sources on peroxyacetyl nitrate (PAN) formation in eastern China in winter, J. Environ. Sci.-China, 94, 81–87, https://doi.org/10.1016/j.jes.2020.03.039, 2020.
Zhang, J. M., Wang, T., Ding, A. J., Zhou, X. H., Xue, L. K., Poon, C. N., Wu, W. S., Gao, J., Zuo, H. C., Chen, J. M., Zhang, X. C., and Fan, S. J.: Continuous measurement of peroxyacetyl nitrate (PAN) in suburban and remote areas of western China, Atmos. Environ., 43, 228–237, https://doi.org/10.1016/j.atmosenv.2008.09.070, 2009.
Zhu, J., Wang, S., Wang, H., Jing, S., Lou, S., Saiz-Lopez, A., and Zhou, B.: Observationally constrained modeling of atmospheric oxidation capacity and photochemical reactivity in Shanghai, China, Atmos. Chem. Phys., 20, 1217–1232, https://doi.org/10.5194/acp-20-1217-2020, 2020.