Papers by Ioannis Tsamardinos
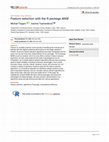
F1000Research
Feature (or variable) selection is the process of identifying the minimal set of features with th... more Feature (or variable) selection is the process of identifying the minimal set of features with the highest predictive performance on the target variable of interest. Numerous feature selection algorithms have been developed over the years, but only few have been implemented in R as a package. The R package MXM is such an example, which not only offers a variety of feature selection algorithms, but has unique features that make it advantageous over its competitors: a) it contains feature selection algorithms that can treat numerous types of target variables, including continuous, percentages, time to event (survival), binary, nominal, ordinal, clustered, counts, left censored, etc; b) it contains a variety of regression models to plug into the feature selection algorithms; c) it includes an algorithm for detecting multiple solutions (many sets of equivalent features); and d) it includes memory efficient algorithms for high volume data, data that cannot be loaded into R. In this paper we qualitatively compare MXM with other relevant packages and discuss its advantages and disadvantages. We also provide a demonstration of its algorithms using real high-dimensional data from various applications.

BMC Bioinformatics, 2018
Background: Feature selection is commonly employed for identifying collectively-predictive biomar... more Background: Feature selection is commonly employed for identifying collectively-predictive biomarkers and biosignatures; it facilitates the construction of small statistical models that are easier to verify, visualize, and comprehend while providing insight to the human expert. In this work we extend established constrained-based, feature-selection methods to high-dimensional " omics " temporal data, where the number of measurements is orders of magnitude larger than the sample size. The extension required the development of conditional independence tests for temporal and/or static variables conditioned on a set of temporal variables. Results: The algorithm is able to return multiple, equivalent solution subsets of variables, scale to tens of thousands of features, and outperform or be on par with existing methods depending on the analysis task specifics. Conclusions: The use of this algorithm is suggested for variable selection with high-dimensional temporal data.
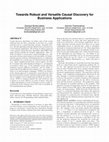
Causal discovery algorithms can induce some of the causal relations from the data, commonly in th... more Causal discovery algorithms can induce some of the causal relations from the data, commonly in the form of a causal network such as a causal Bayesian network. Arguably however , all such algorithms lack far behind what is necessary for a true business application. We develop an initial version of a new, general causal discovery algorithm called ETIO with many features suitable for business applications. These include (a) ability to accept prior causal knowledge (e.g., taking senior driving courses improves driving skills), (b) admitting the presence of latent confounding factors, (c) admitting the possibility of (a certain type of) selection bias in the data (e.g., clients sampled mostly from a given region), (d) ability to analyze data with missing-by-design (i.e., not planned to measure) values (e.g., if two companies merge and their databases measure different attributes), and (e) ability to analyze data from different interventions (e.g., prior and posterior to an advertisement campaign). ETIO is an instance of the logical approach to integrative causal discovery that has been relatively recently introduced and enables the solution of complex reverse-engineering problems in causal discovery. ETIO is compared against the state-of-the-art and is shown to be more effective in terms of speed, with only a slight degradation in terms of learning accuracy, while incorporating all the features above.The code is available on the mensxmachina.org website.
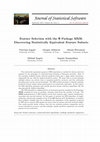
The statistically equivalent signature (SES) algorithm is a method for feature selection inspired... more The statistically equivalent signature (SES) algorithm is a method for feature selection inspired by the principles of constrained-based learning of Bayesian Networks. Most of the currently available feature-selection methods return only a single subset of features, supposedly the one with the highest predictive power. We argue that in several domains multiple subsets can achieve close to maximal predictive accuracy, and that arbitrarily providing only one has several drawbacks. The SES method attempts to identify multiple, predictive feature subsets whose performances are statistically equivalent. Under that respect SES subsumes and extends previous feature selection algorithms, like the max-min parent children algorithm. SES is implemented in an homonym function included in the R package MXM, standing for mens ex machina, meaning 'mind from the machine' in Latin. The MXM implementation of SES handles several data-analysis tasks, namely classification, regression and survival analysis. In this paper we present the SES algorithm, its implementation, and provide examples of use of the SES function in R. Furthermore, we analyze three publicly available data sets to illustrate the equivalence of the signatures retrieved by SES and to contrast SES against the state-of-the-art feature selection method LASSO. Our results provide initial evidence that the two methods perform comparably well in terms of pre-dictive accuracy and that multiple, equally predictive signatures are actually present in real world data.
2008 Computers in Cardiology, 2008
Significant clinical information can be obtained from the analysis of the dominant beat morpholog... more Significant clinical information can be obtained from the analysis of the dominant beat morphology. In such respect, the identification of the dominant beats and their averaging can be very helpful, allowing clinicians to perform the measurement of amplitudes and intervals on a beat much cleaner from noise than a generic beat selected from the entire ECG recording.
Cancer informatics
Sound data analysis is critical to the success of modern molecular medicine research that involve... more Sound data analysis is critical to the success of modern molecular medicine research that involves collection and interpretation of mass-throughput data. The novel nature and high-dimensionality in such datasets pose a series of nontrivial data analysis problems. This technical commentary discusses the problems of over-fi tting, error estimation, curse of dimensionality, causal versus predictive modeling, integration of heterogeneous types of data, and lack of standard protocols for data analysis. We attempt to shed light on the nature and causes of these problems and to outline viable methodological approaches to overcome them.

Journal of Machine Learning Research
In part I of this work we introduced and evaluated the Generalized Local Learning (GLL) framework... more In part I of this work we introduced and evaluated the Generalized Local Learning (GLL) framework for producing local causal and Markov blanket induction algorithms. In the present second part we analyze the behavior of GLL algorithms and provide extensions to the core methods. Specifically, we investigate the empirical convergence of GLL to the true local neighborhood as a function of sample size. Moreover, we study how predictivity improves with increasing sample size. Then we investigate how sensitive are the algorithms to multiple statistical testing, especially in the presence of many irrelevant features. Next we discuss the role of the algorithm parameters and also show that Markov blanket and causal graph concepts can be used to understand deviations from optimality of state-of-the-art non-causal algorithms. The present paper also introduces the following extensions to the core GLL framework: parallel and distributed versions of GLL algorithms, versions with false discovery rate control, strategies for constructing novel heuristics for specific domains, and divide-and-conquer local-to-global learning (LGL) strategies. We test the generality of the LGL approach by deriving a novel LGL-based algorithm that compares favorably c 2010 Constantin F. Aliferis, Alexander Statnikov, Ioannis Tsamardinos, Subramani Mani and Xenofon D. Koutsoukos. ALIFERIS, STATNIKOV, TSAMARDINOS, MANI AND KOUTSOUKOS to the state-of-the-art global learning algorithms. In addition, we investigate the use of non-causal feature selection methods to facilitate global learning. Open problems and future research paths related to local and local-to-global causal learning are discussed.

Temporal plans permit significant flexibility in specifying the occurrence time of events. Plan e... more Temporal plans permit significant flexibility in specifying the occurrence time of events. Plan execution can make good use of that flexibility. However, the advantage of execution flexibility is counterbalanced by the cost during execution of propagating the time of occurrence of events throughout the flexible plan. To minimize execution latency, this propagation needs to be very efficient. Previous work showed that every temporal plan can be reformulated as a dispatchable plan, i.e., one for which propagation to immediate neighbors is sufficient. A simple algorithm was given that finds a dispatchable plan with a minimum number of edges in cubic time and quadratic space. In this paper, we focus on the efficiency of the reformulation process, and improve on that result. A new algorithm is presented that uses linear space and has time complexity equivalent to Johnson's algorithm for all-pairs shortest-path problems. Experimental evidence confirms the practical effectiveness of the new algorithm. For example, on a large commercial application, the performance is improved by at least two orders of magnitude. We further show that the dispatchable plan, already minimal in the total number of edges, can also be made minimal in the maximum number of edges incoming or outgoing at any node. *
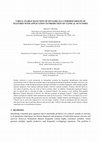
Feature selection is used extensively in biomedical research for biomarker identification and pat... more Feature selection is used extensively in biomedical research for biomarker identification and patient classification, both of which are essential steps in developing personalized medicine strategies. However, the structured nature of the biological datasets and high correlation of variables frequently yield multiple equally optimal signatures, thus making traditional feature selection methods unstable. Features selected based on one cohort of patients, may not work as well in another cohort. In addition, biologically important features may be missed due to selection of other co-clustered features We propose a new method, Tree-guided Recursive Cluster Selection (T-ReCS), for efficient selection of grouped features. T-ReCS significantly improves predictive stability while maintains the same level of accuracy. T-ReCS does not require an a priori knowledge of the clusters like group-lasso and also can handle "orphan" features (not belonging to a cluster). T-ReCS can be used wi...
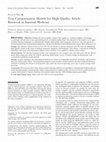
Journal of the American Medical Informatics Association
OBJECTIVE Finding the best scientific evidence that applies to a patient problem is becoming exce... more OBJECTIVE Finding the best scientific evidence that applies to a patient problem is becoming exceedingly difficult due to the exponential growth of medical publications. The objective of this study was to apply machine learning techniques to automatically identify high-quality, content-specific articles for one time period in internal medicine and compare their performance with previous Boolean-based PubMed clinical query filters of Haynes et al. DESIGN The selection criteria of the ACP Journal Club for articles in internal medicine were the basis for identifying high-quality articles in the areas of etiology, prognosis, diagnosis, and treatment. Naive Bayes, a specialized AdaBoost algorithm, and linear and polynomial support vector machines were applied to identify these articles. MEASUREMENTS The machine learning models were compared in each category with each other and with the clinical query filters using area under the receiver operating characteristic curves, 11-point average ...

Temporal uncertainty is a feature of many real-world planning problems. One of the most successfu... more Temporal uncertainty is a feature of many real-world planning problems. One of the most successful formalisms for dealing with temporal uncertainty is the Simple Temporal Problem with uncertainty (STP-u). A very attractive feature of STP-u's is that one can determine in polynomial time whether a given STP-u is dynamically controllable, i.e., whether there is a guaranteed means of execution such that all the constraints are respected, regardless of the exact timing of the uncertain events. Unfortunately, if the STP-u is not dynamically controllable, limitations of the formalism prevent further reasoning about the probability of legal execution. In this paper, we present an alternative formalism, called Probabilistic Simple Temporal Problems (PSTPs), which generalizes STP-u to allow for such reasoning. We show that while it is difficult to compute the exact probability of legal execution, there are methods for bounding the probability both from above and below, and we sketch alter...
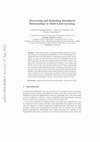
This work presents a sound probabilistic method for enforcing adherence of the marginal probabili... more This work presents a sound probabilistic method for enforcing adherence of the marginal probabilities of a multi-label model to automatically discovered deterministic relationships among labels. In particular we focus on discovering two kinds of relationships among the labels. The first one concerns pairwise positive entailement: pairs of labels, where the presence of one implies the presence of the other in all instances of a dataset. The second concerns exclusion: sets of labels that do not coexist in the same instances of the dataset. These relationships are represented with a Bayesian network. Marginal probabilities are entered as soft evidence in the network and adjusted through probabilistic inference. Our approach offers robust improvements in mean average precision compared to the standard binary relavance approach across all 12 datasets involved in our experiments. The discovery process helps interesting implicit knowledge to emerge, which could be useful in itself.

The Markov blanket of a target variable is the minimum conditioning set of variables that makes t... more The Markov blanket of a target variable is the minimum conditioning set of variables that makes the target independent of all other variables. Markov blankets inform feature selection, aid in causal discovery and serve as a basis for scalable methods of constructing Bayesian networks. We apply decision tree induction to the task of Markov blanket identification. Notably, we compare (a) C5.0, a widely used algorithm for decision rule induction, (b) C5C, which post-processes C5.0 's rule set to retain the most frequently referenced variables and (c) PC, a standard method for Bayesian network induction. C5C performs as well as or better than C5.0 and PC across a number of data sets. Our modest variation of an inexpensive, accurate, off-the-shelf induction engine mitigates the need for specialized procedures, and establishes baseline performance against which specialized algorithms can be compared.
Communications in Computer and Information Science, 2013

Lecture Notes in Computer Science, 2002
In Temporal Planning a typical assumption is that the agent controls the execu- tion time of all ... more In Temporal Planning a typical assumption is that the agent controls the execu- tion time of all events such as starting and ending actions. In real domains how- ever, this assumption is commonly violated and certain events are beyond the di- rect control of the plan's executive. Previous work on reasoning with uncontrol- lable events (Simple Temporal Problem with Uncertainty) assumes that we can bound the occurrence of each uncontrollable within a time interval. In principle however, there is no such bounding interval since there is always a non-zero probability the event will occur outside the bounds. Here we develop a new more general formalism called the Probabilistic Simple Temporal Problem (PSTP) fol- lowing a probabilistic approach. We present a method for scheduling a PSTP maximizing the probability of correct execution. Subsequently, we use this method to solve the problem of finding an optimal execution strategy, i.e. a dy- namic schedule where scheduling decisions can be made on-line.

Lecture Notes in Computer Science, 2002
In this paper we discuss our work on plan management in the Autominder cognitive orthotic system.... more In this paper we discuss our work on plan management in the Autominder cognitive orthotic system. Autominder is being designed as part of an initiative on the development of robotic assistants for the elderly. Autominder stores and updates user plans, tracks their execution via input from robot sensors, and provides carefully chosen and timed reminders of the activities to be performed. It will eventually also learn the typical behavior of the user with regard to the execution of these plans. A central component of Autominder is its Plan Manager (PM), which is responsible for the temporal reasoning involved in updating plans and tracking their execution. The PM models plan update problems as disjunctive temporal problems (DTPs) and uses the Epilitis DTPsolving system to handle them. We describe the plan representations and algorithms used by the Plan Manager, and briefly discuss its connections with the rest of the system.

PLOS ONE, 2015
We address the problem of predicting the position of a miRNA duplex on a microRNA hairpin via the... more We address the problem of predicting the position of a miRNA duplex on a microRNA hairpin via the development and application of a novel SVM-based methodology. Our method combines a unique problem representation and an unbiased optimization protocol to learn from mirBase19.0 an accurate predictive model, termed MiRduplexSVM. This is the first model that provides precise information about all four ends of the miRNA duplex. We show that (a) our method outperforms four state-of-the-art tools, namely MaturePred, MiRPara, MatureBayes, MiRdup as well as a Simple Geometric Locator when applied on the same training datasets employed for each tool and evaluated on a common blind test set. (b) In all comparisons, MiRduplexSVM shows superior performance, achieving up to a 60% increase in prediction accuracy for mammalian hairpins and can generalize very well on plant hairpins, without any special optimization. (c) The tool has a number of important applications such as the ability to accurately predict the miRNA or the miRNA*, given the opposite strand of a duplex. Its performance on this task is superior to the 2nts overhang rule commonly used in computational studies and similar to that of a comparative genomic approach, without the need for prior knowledge or the complexity of performing multiple alignments. Finally, it is able to evaluate novel, potential miRNAs found either computationally or experimentally. In relation with recent confidence evaluation methods used in miRBase, MiRduplexSVM was successful in identifying high confidence potential miRNAs.
Uploads
Papers by Ioannis Tsamardinos