This article has been reviewed according to Science X's editorial process and policies. Editors have highlighted the following attributes while ensuring the content's credibility:
fact-checked
peer-reviewed publication
trusted source
proofread
Scientists create scalable solution for analyzing single-cell data
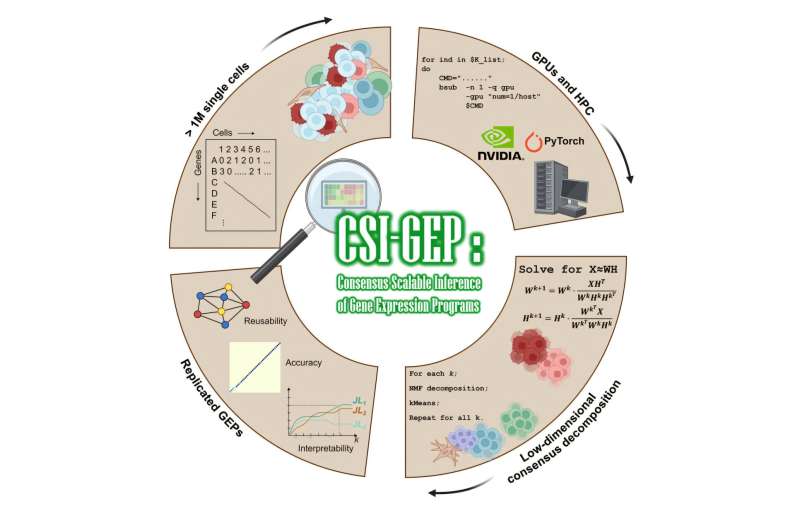
Researchers have amassed vast single-cell gene expression databases to understand how the smallest details impact human biology. However, current analysis methods struggle with the large volume of data and, as a result, produce biased and contradictory findings.
Scientists at St. Jude Children's Research Hospital created a machine-learning algorithm capable of scaling with these single-cell data repositories to deliver more accurate results. The new method was published today (Jan. 8) in Cell Genomics.
Before single-cell analysis, bulk gene expression data gave high-level but unrefined results for many diseases. Single-cell analysis enables researchers to look at individual cells of interest, a difference akin to looking at an individual corn kernel instead of a field. These detailed insights have already made breakthroughs in understanding some diseases and treatments, but difficulty replicating and scaling analyses for data that keeps increasing in size has stymied progress.
"We've implemented a new toolset that can be scaled as these single-cell RNA sequencing datasets continue to grow," said corresponding author Paul Geeleher, Ph.D., St. Jude Department of Computational Biology. "There has been an exponential explosion in the compute time for single-cell analysis, and our method brings accurate analysis back into a tractable timeframe."
All techniques for studying single-cell gene expression create large amounts of data. When scientists test millions of cells simultaneously, the amount of computer memory and processing power needed to handle the data is enormous. Geeleher's team turned to a different kind of hardware to help solve the problem.
"We created a method that uses graphics processing units or GPUs," said first author Xueying Liu, Ph.D., St. Jude Department of Computational Biology. "The GPU integration gave us the processing power to perform the computational load in a scalable way."
Unsupervised machine learning for single-cell analysis
The volume of data often forces researchers to make concessions and assumptions that introduce biases when conducting analyses with standard methods. The St. Jude scientists used an artificial intelligence approach that removes such bias from these selections.
"Our method uses unsupervised machine learning, which automatically determines more robust and less arbitrary parameters for the analysis," Liu said. "It learns how to group cells based on their different active biological processes or cell type identities."
Since the algorithm learns and derives its analysis from the data presented, researchers could use it on any sizeable single-cell RNA sequencing dataset. As it investigates each new large dataset individually and only uses those expression program clues to make conclusions, the researchers called the approach the Consensus and Scalable Inference of Gene Expression Programs (CSI-GEP).
When applied to the largest single-cell RNA databases, CSI-GEP produced better results than every other method. Most impressively, the algorithm could identify cell types and the activity of biological processes missed by other methods.
"We've created a tool broadly applicable to studying any disease through single-cell RNA analysis," Geeleher said. "The method performed substantially better than all existing approaches we tested, so I hope other scientists consider using it to get better value out of their single-cell data."
CSI-GEP is freely available on GitHub.
More information: Xueying Liu et al, CSI-GEP: A GPU-based unsupervised machine learning approach for recovering gene expression programs in atlas-scale single-cell RNA-seq data, Cell Genomics (2025). DOI: 10.1016/j.xgen.2024.100739
Journal information: Cell Genomics
Provided by St. Jude Children's Research Hospital